Homology-Class Guided Rapidly-Exploring Random Tree For Belief Space Planning
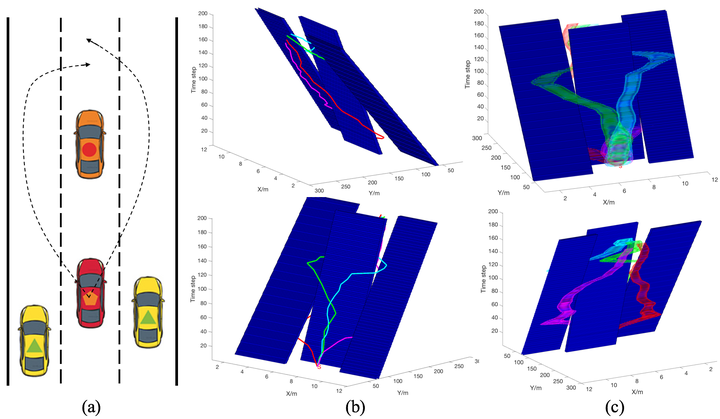
In this work, an efficient homology guided belief space planning method for obstacle-cluttered environments is presented. The proposed planner follows a two-step approach. First, a h-signature guided rapidly-exploring random tree (HRRT) algorithm is proposed to provide nominal trajectories in different homology classes by constructing homology aware sub-trees in a parallel manner. The HRRT planner is extended to a h-signature guided RRT* algorithm, where an inter-homology-class rewire procedure is proposed, increasing the probability of discovering homology classes in narrow space/passages. The iLQG-based belief space planning algorithm is then employed to find locally optimal trajectories minimizing uncertainties in each homology class.